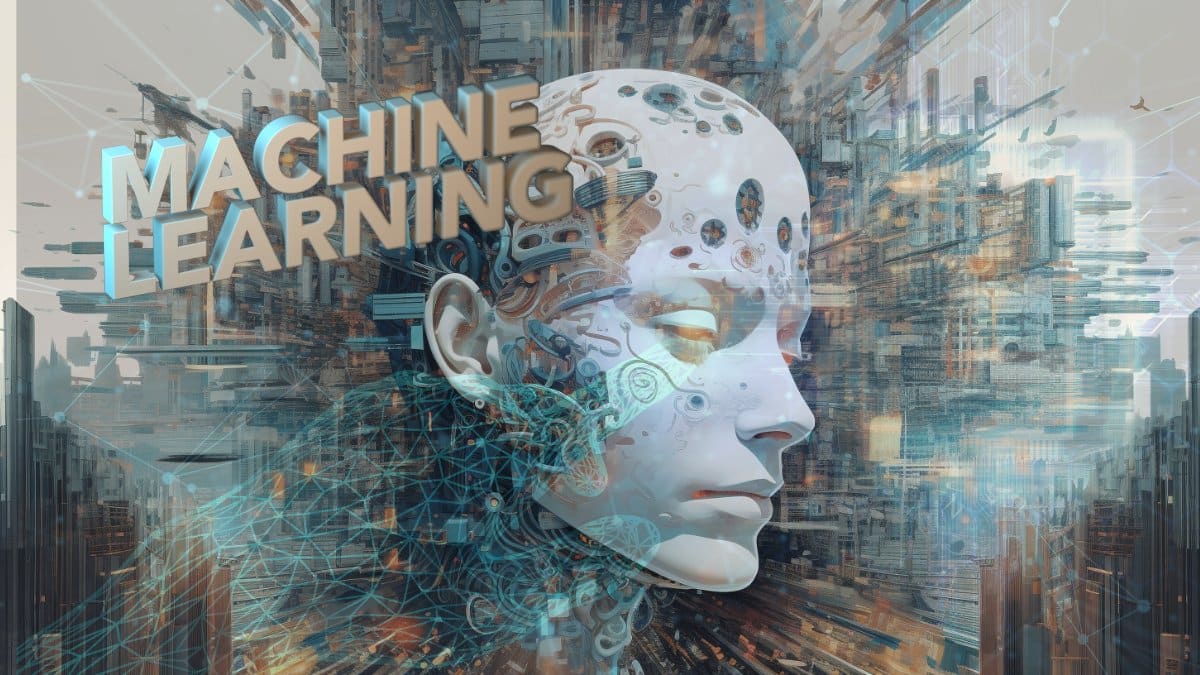
May 29, 2023
unlocking the power of machine learning in 2023
Imagine a world where computers can predict your needs, make recommendations based on your preferences, and even diagnose diseases more accurately than humans. This future is already here thanks to the power of machine learning. In this ever-evolving technological landscape, machine learning is transforming industries and becoming an indispensable tool for businesses, governments, and individuals alike. Unlocking machine learning ml all its potential requires understanding the basics, real-world applications, challenges, and future trends. Are you ready to dive into the fascinating world of machine learning? Let's begin!,- Machine learning is an essential tool for deriving meaningful insights from data and experience. ,- It has been utilized in both governmental and business contexts to optimize decision-making, improve public services, and offer practical solutions to complex problems. ,- The near future of machine learning is set to be characterized by ethical AI & responsible innovation with increased access to efficient tools., ,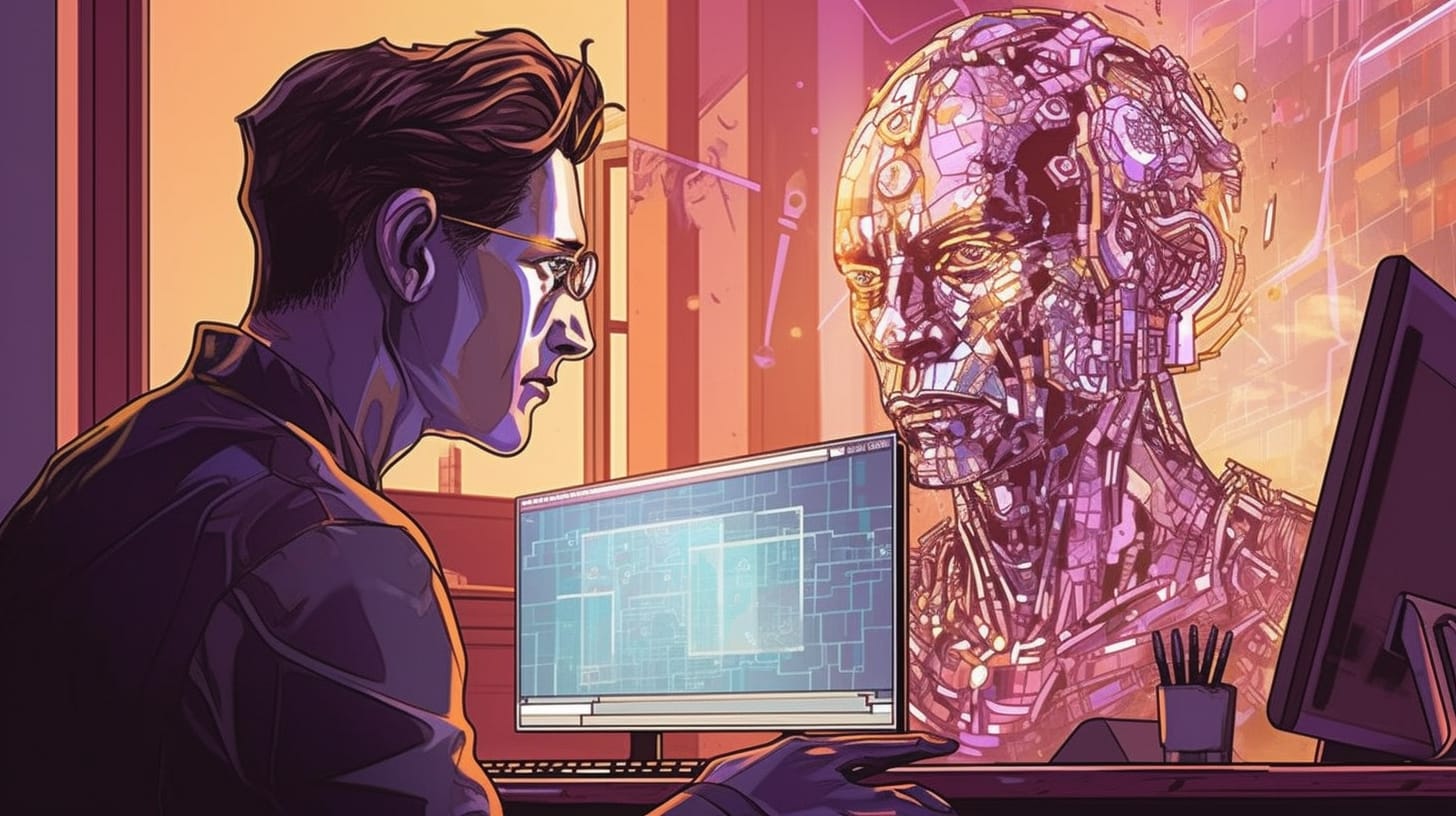,Machine learning, a type of artificial intelligence, empowers computers to learn from data and experience without the need for explicit programming. The concept has been around since the 1950s when Alan Turing submitted a groundbreaking paper that laid the foundation for machine learning.,Today, with advances in computing power and data availability, machine learning has become an essential tool for solving complex problems and providing valuable insights.,In essence, machine learning is the process that allows machines to learn autonomously and produce outputs without explicit programming. It follows an approach where computers learn to program themselves through experience. Machine learning can be categorized into four main types: supervised learning, unsupervised learning, semi-supervised learning, and reinforcement learning.,Supervised unsupervised machine learning, the most common type, involves training an algorithm using labeled data to make predictions about new, unseen data. In contrast, unsupervised learning deals with unlabeled data and finds hidden patterns or structures within the data.,Semi-supervised learning combines elements of both supervised and unsupervised learning, using a smaller set of labeled data and a larger set of unlabeled and labeled training data together. Lastly, reinforcement learning focuses on teaching algorithms to make decisions by interacting with an environment and receiving feedback in the form of rewards or penalties.,Machine learning is a technique employed to utilize artificial intelligence (AI). AI aims to construct computer models that demonstrate "intelligent behaviors" comparable to those of humans, such as recognizing visual scenes, understanding natural language, and executing actions in the physical world. Machine learning plays a crucial role in enabling AI systems to learn from data and make decisions based on algorithms.,Deep learning, a subset of machine learning, is computer science that involves the development of artificial neural networks that can process data and make decisions. These networks are designed to mimic the way the human brain works, enabling machines to perform tasks with high levels of accuracy and efficiency.,As technology advances, the relationship between machine learning, AI, and deep learning will continue to strengthen, leading to more sophisticated and powerful AI systems.,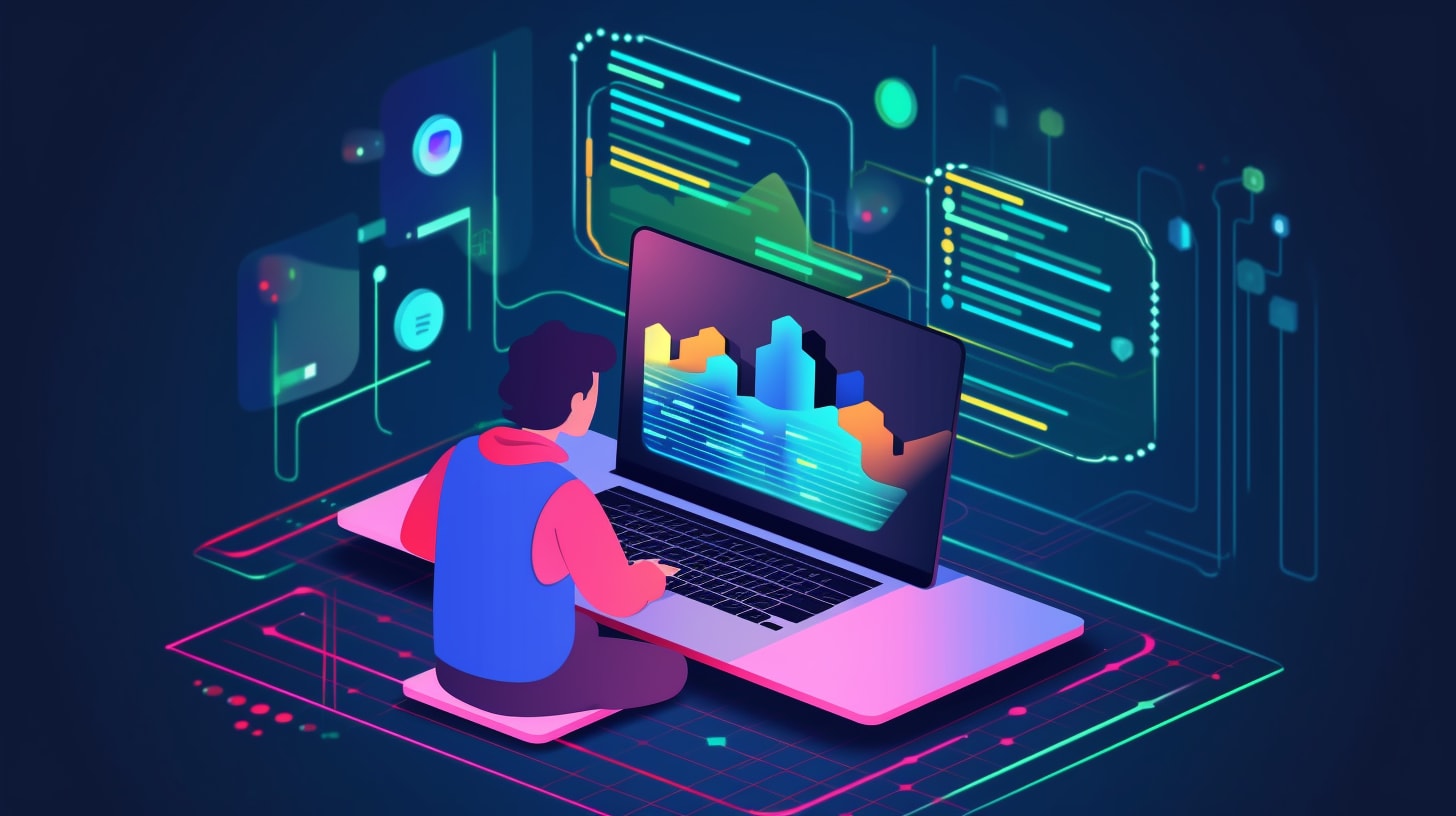,Machine learning has found its way into various aspects of our lives, offering solutions to complex problems and enhancing decision-making capabilities. Governments use machine learning to analyze citizens' interests and needs, allowing them to better allocate resources and improve public services.,Businesses leverage machine learning for fraud detection, spam filtering, business process automation, and predictive maintenance, among other applications.,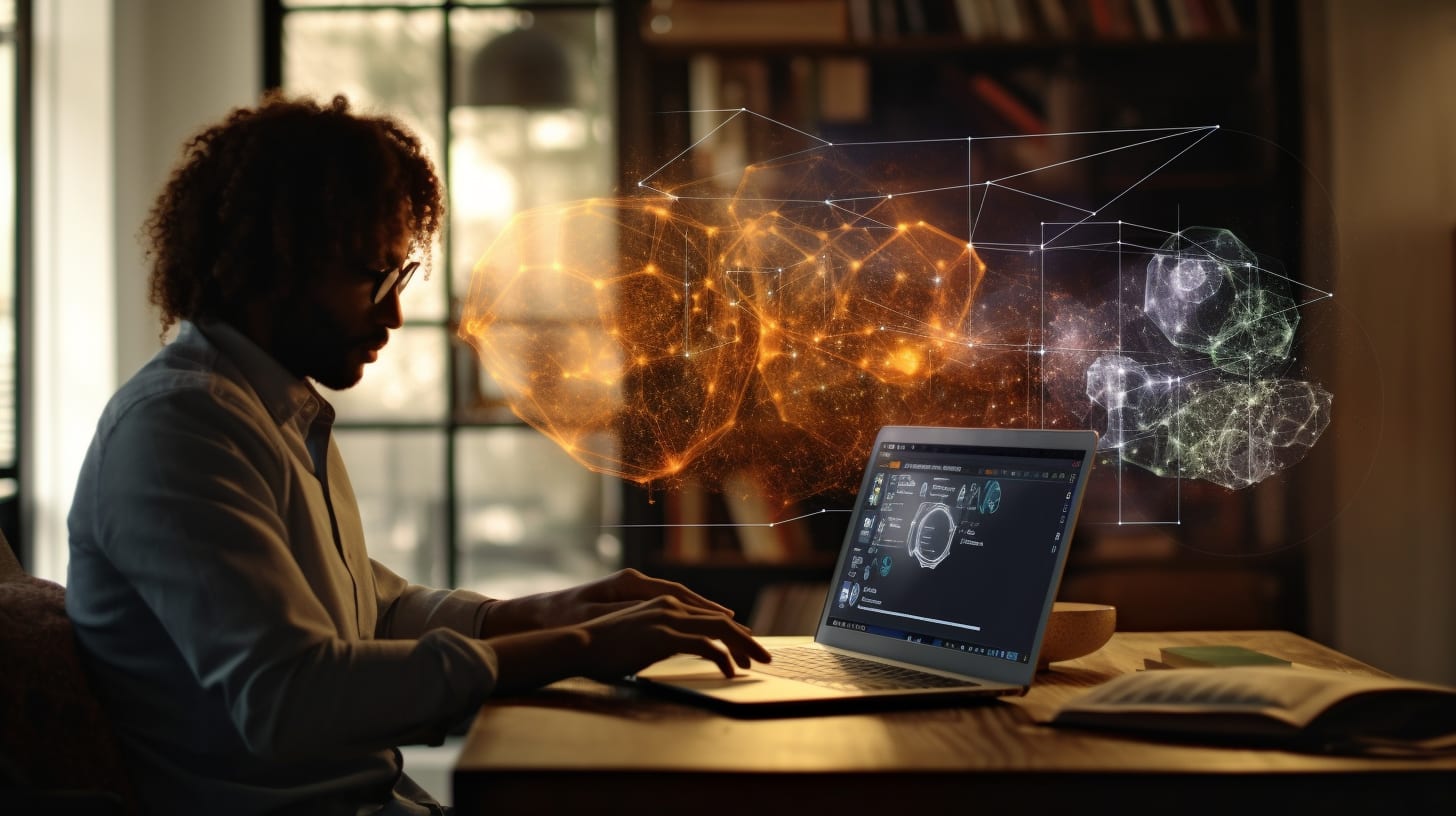,One of the most recognizable applications of machine learning is in personalization and recommendation algorithms. These algorithms power the suggestions we see on platforms like Netflix, YouTube, and Facebook, adapting to our preferences and behavior to provide content that is most relevant to us. Online retailers also use machine learning for merchandise supply planning and targeted marketing campaigns, personalizing the shopping experience for each customer.,As machine learning algorithms become more advanced, they are able to better understand and predict our preferences, leading to a more personalized and efficient user experience. In the future, we can expect even greater levels of personalization across various industries, as machine learning continues to improve and evolve.,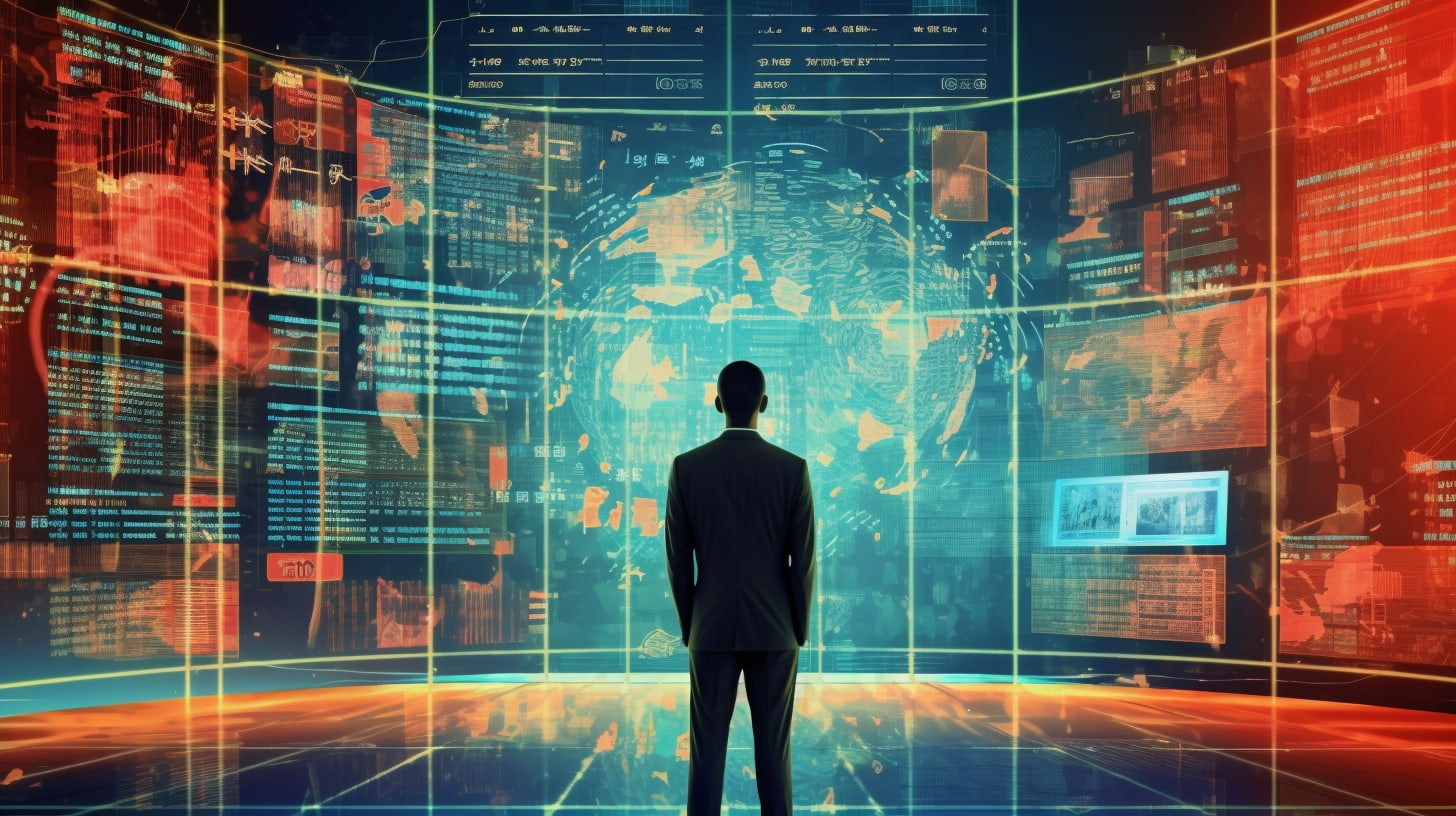,Image and speech pattern recognition are other prominent applications of machine learning. Image recognition involves classifying pictures based on their intensity or measurement of intensities of red, blue, and green for colored images. Speech recognition, on the other hand, translates spoken words into text and segments them according to their frequency. These techniques are widely used in facial recognition, voice recognition, and automated customer service systems.,Machine learning has significantly improved the accuracy and efficiency of image and speech recognition systems. As technology progresses, we can expect even more advanced capabilities in this domain, with potential use cases in security, healthcare, and other industries.,Machine learning is revolutionizing the healthcare and pharmaceutical industries by improving disease diagnosis, enabling personalized treatment, and accelerating drug discovery. By analyzing large amounts of medical data, machine learning algorithms can identify patterns and trends that would be difficult for humans to detect, leading to more accurate and timely diagnoses.,In the pharmaceutical industry, supervised machine learning can reduce costs and obtain precise outcomes in drug design and testing by comparing data of drugs and their chemical compounds with various parameters. As the machine learning technology continues to advance, its impact on healthcare and pharmaceuticals is expected to grow, ultimately leading to better patient outcomes and more efficient healthcare systems.,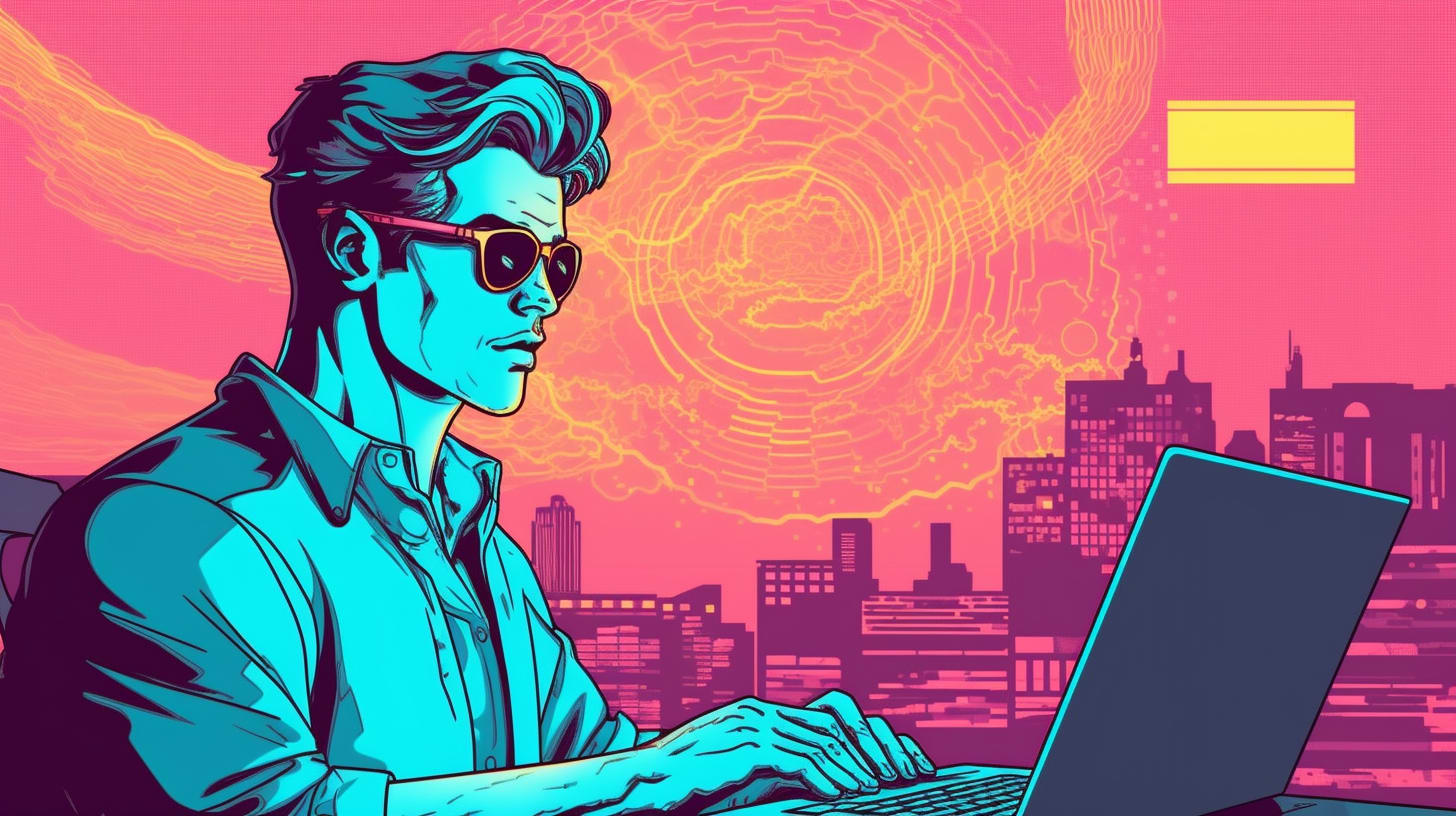,As supervised machine learning becomes more prevalent, it is crucial to address the challenges and ethical considerations associated with its use. Ensuring that AI technologies are employed responsibly and ethically is of paramount importance, as the implications of these technologies can be far-reaching and have significant consequences.,Some of the main challenges in this area include bias and discrimination, privacy and security, and explainability and interpretability.,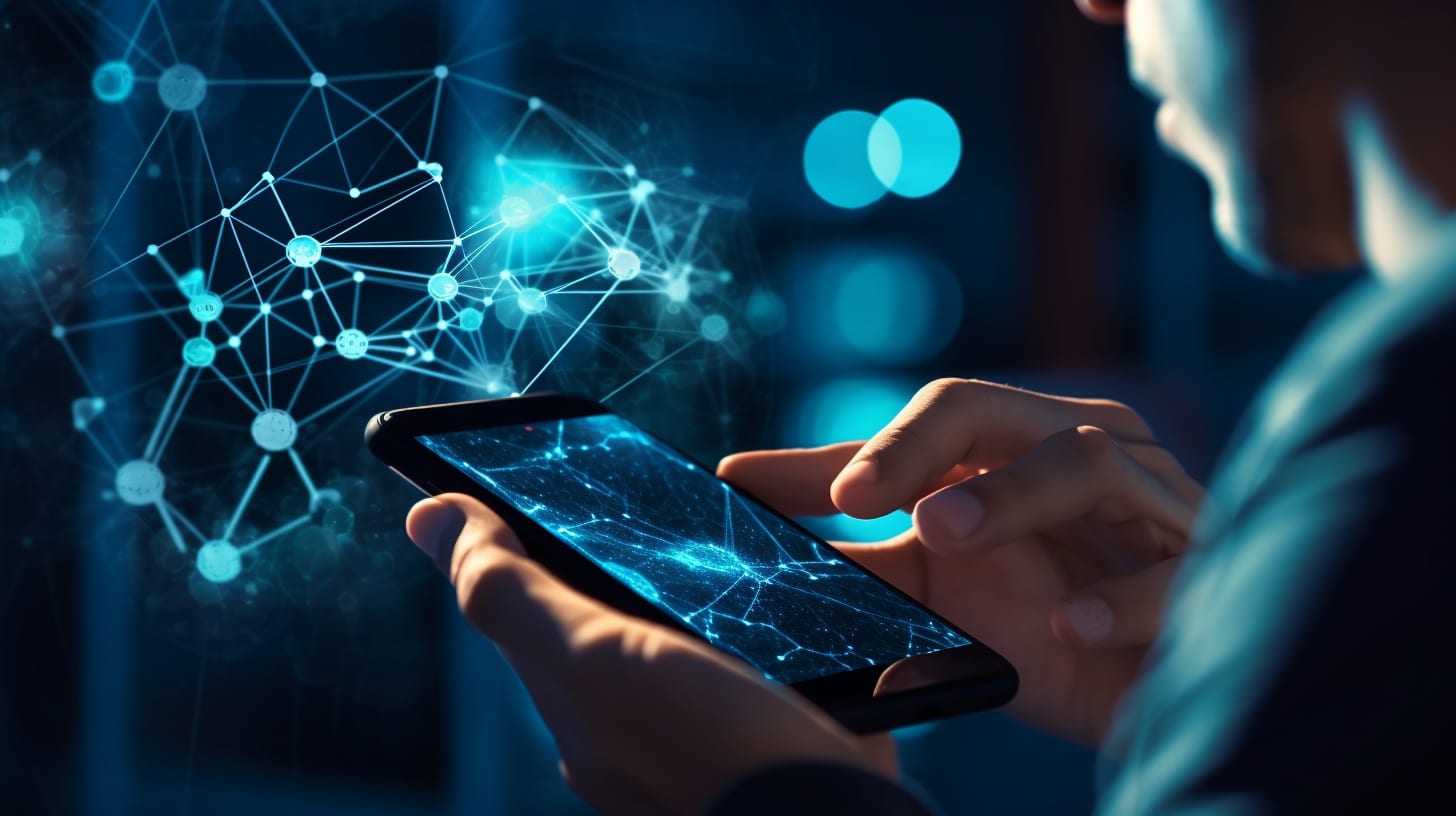,Bias in machine learning occur when an algorithm produces systematically prejudiced results due to erroneous assumptions or the use of biased data during the learning process. This can lead to discrimination against certain groups or individuals, perpetuating existing social inequalities. Bias in a machine learning algorithm can manifest in various ways, such as data collection and labeling, data representation, and algorithmic bias.,To mitigate the risk of bias and discrimination, it is essential to use representative and unbiased data sets for training machine learning algorithms. Additionally, the development of transparent and accountable algorithms can help ensure that machine learning systems are equitable and fair.,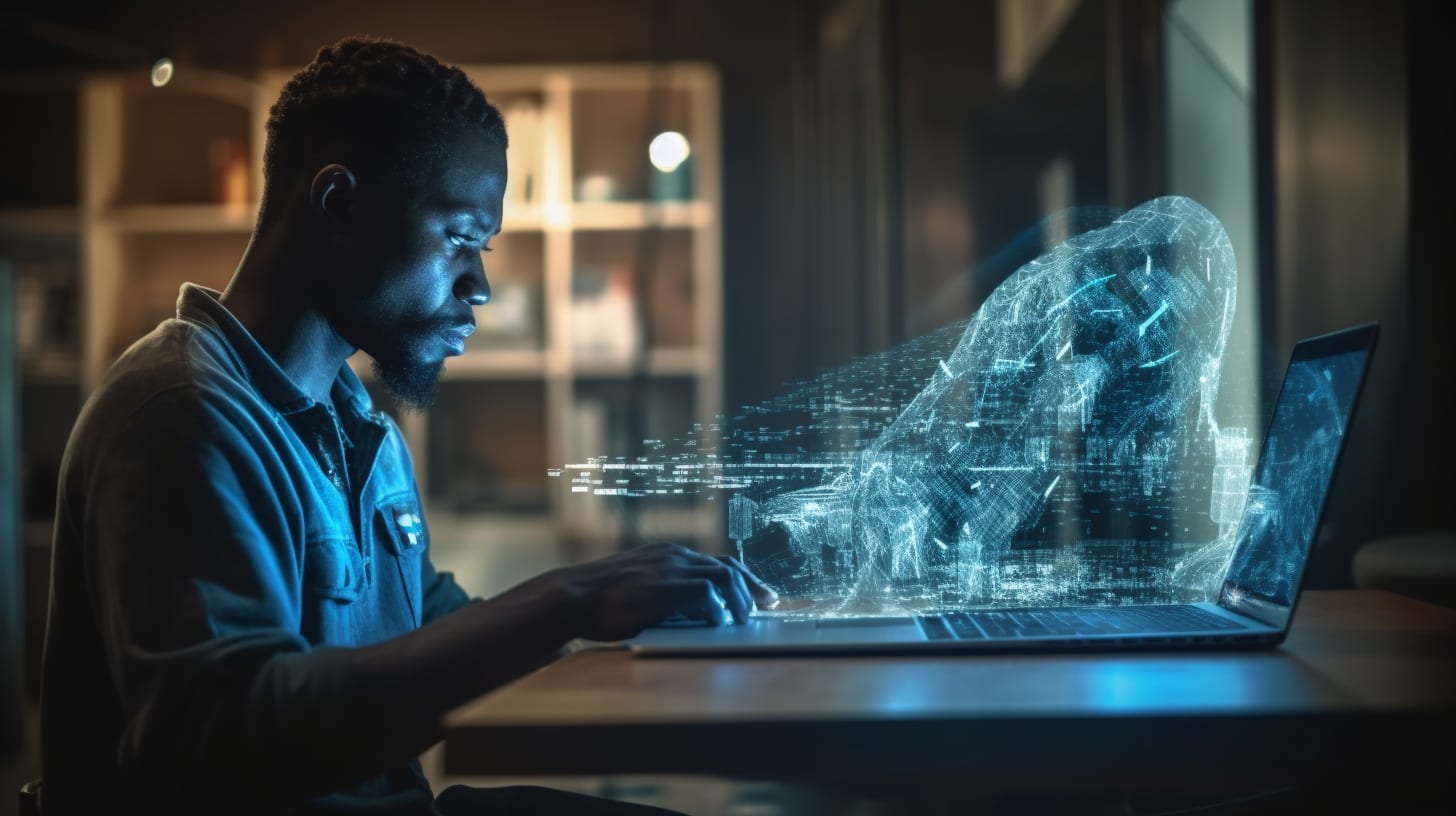,Privacy and security are critical concerns in the field of machine learning. Ensuring the protection of sensitive data used in the training or prediction process is essential, as is guaranteeing that the output of the model does not reveal private information about individuals.,Furthermore, machine learning models can be susceptible to malicious attacks, which can lead to inaccurate results and erroneous decisions. To address these challenges, techniques such as differential privacy and privacy-preserving machine and supervised learning algorithms can be employed to protect user data and maintain privacy.,Additionally, implementing robust security measures to safeguard data and machine learning models is crucial to prevent unauthorized access and potential abuse.,Explainability and interpretability in machine learning refer to the ability to provide clarity regarding the operations and decision-making processes of machine learning models. Understanding why a model produces certain outputs and how it arrives at those decisions can be a complex task, but it is essential to ensure the model's validity and trustworthiness.,Techniques such as explainable AI can be used to make the decision-making process of machine learning algorithms more comprehensible and interpretable, allowing stakeholders to better understand and trust the model's outcomes. Ensuring explainability and interpretability is particularly important in industries with stringent compliance requirements, such as banking and insurance.,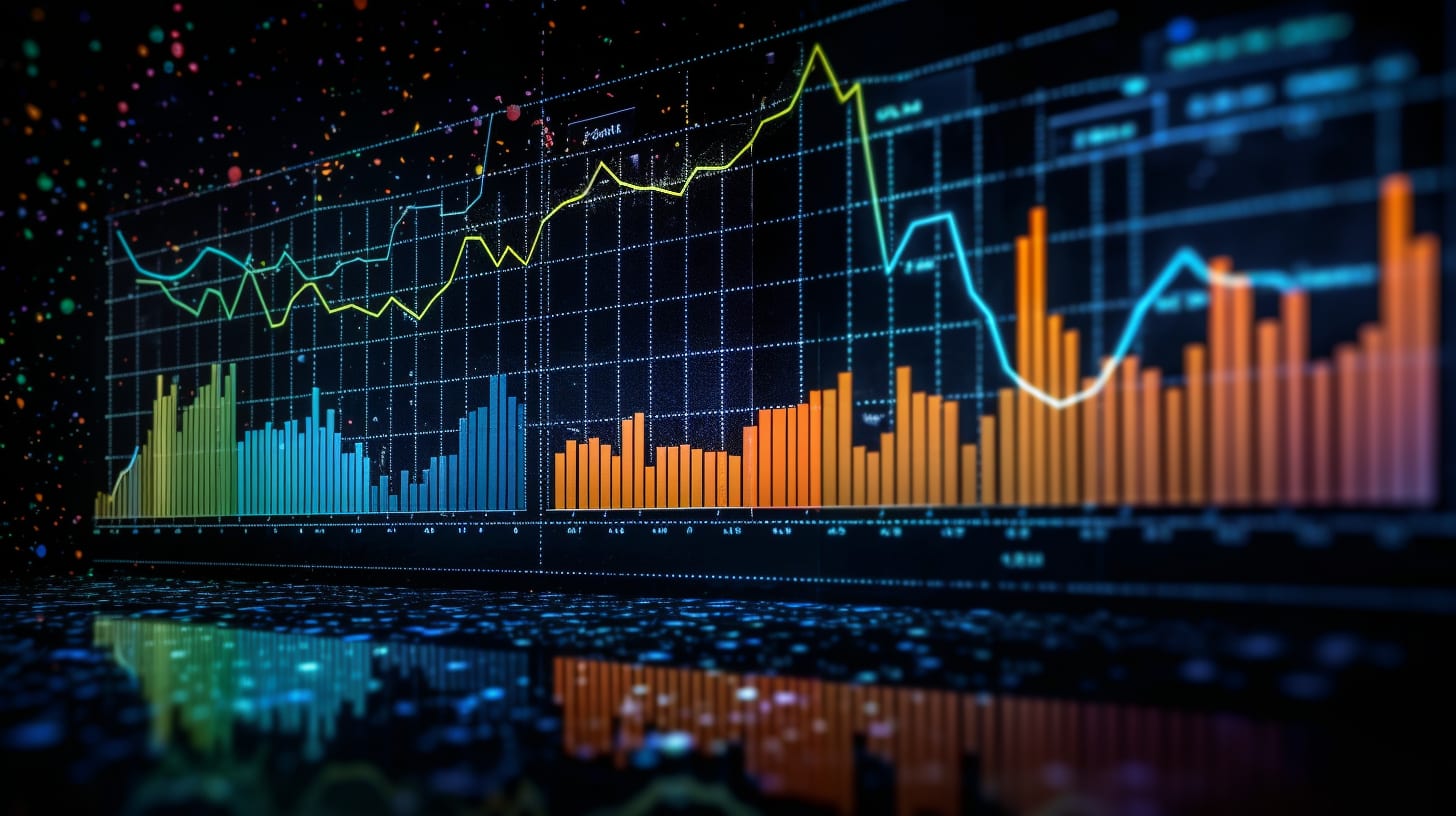,To effectively implement machine learning in business, executives must first identify a business problem or customer need that could be addressed through machine learning. This requires a fundamental understanding of machine learning concepts and techniques, as well as the collaboration of people with diverse expertise.,Once a suitable use case is identified, the next steps involve using sample data, selecting the right model and using a collaborative approach to develop and refine the machine learning solution.,Machine learning can address various business problems, ranging from predicting customer behavior and optimizing supply chain management to detecting fraud and refining marketing initiatives. Additionally, it can assist with tasks such as data entry, financial analysis, and predictive maintenance. Prioritizing a business problem that can be effectively addressed with a machine learning system is crucial to maximize its impact and ensure a successful implementation.,By leveraging machine learning to address business challenges, companies can reap numerous benefits, such as improved accuracy, accelerated processing, and enhanced decision-making. Furthermore, machine learning can help reduce costs and increase efficiency, providing a significant competitive advantage.,Selecting the appropriate machine learning model is a crucial step in the implementation process. Factors to consider when choosing the right model include the type of training data used, the complexity of the problem, the accuracy of the model, and the resources available. Machine learning models can be broadly classified into two types: classification and regression. Additionally, there are four main types of machine learning algorithms: supervised, semi-supervised, unsupervised, and reinforcement.,By carefully evaluating these factors and selecting the most suitable model, executives can ensure the success of their machine learning implementation and maximize its impact on their business.,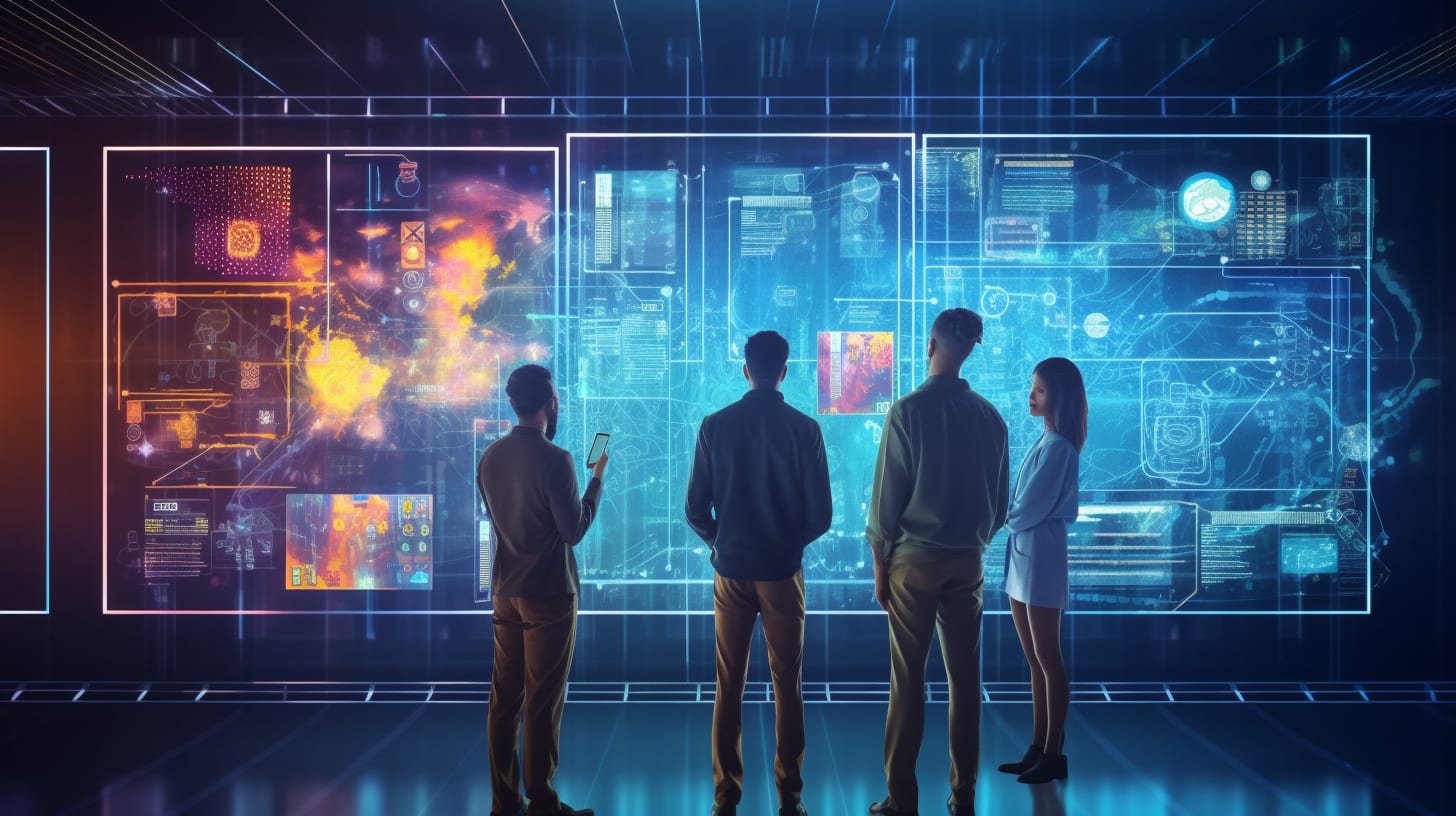,A collaborative approach to machine learning involves multiple parties working together to develop and refine a machine learning model. This can include sharing data, knowledge, and resources to achieve a shared objective. Federated learning, for instance, is a collaborative approach that allows multiple individuals or teams to collectively train a model without exchanging their data, thereby preserving privacy.,The benefits of a collaborative approach to machine learning include cost and time efficiency, as well as improved model accuracy. However, challenges may arise in ensuring that all participants have access to and can utilize the necessary data and resources to create and enhance the model. Addressing these challenges and fostering a collaborative environment can lead to more effective machine learning implementations and better business outcomes.,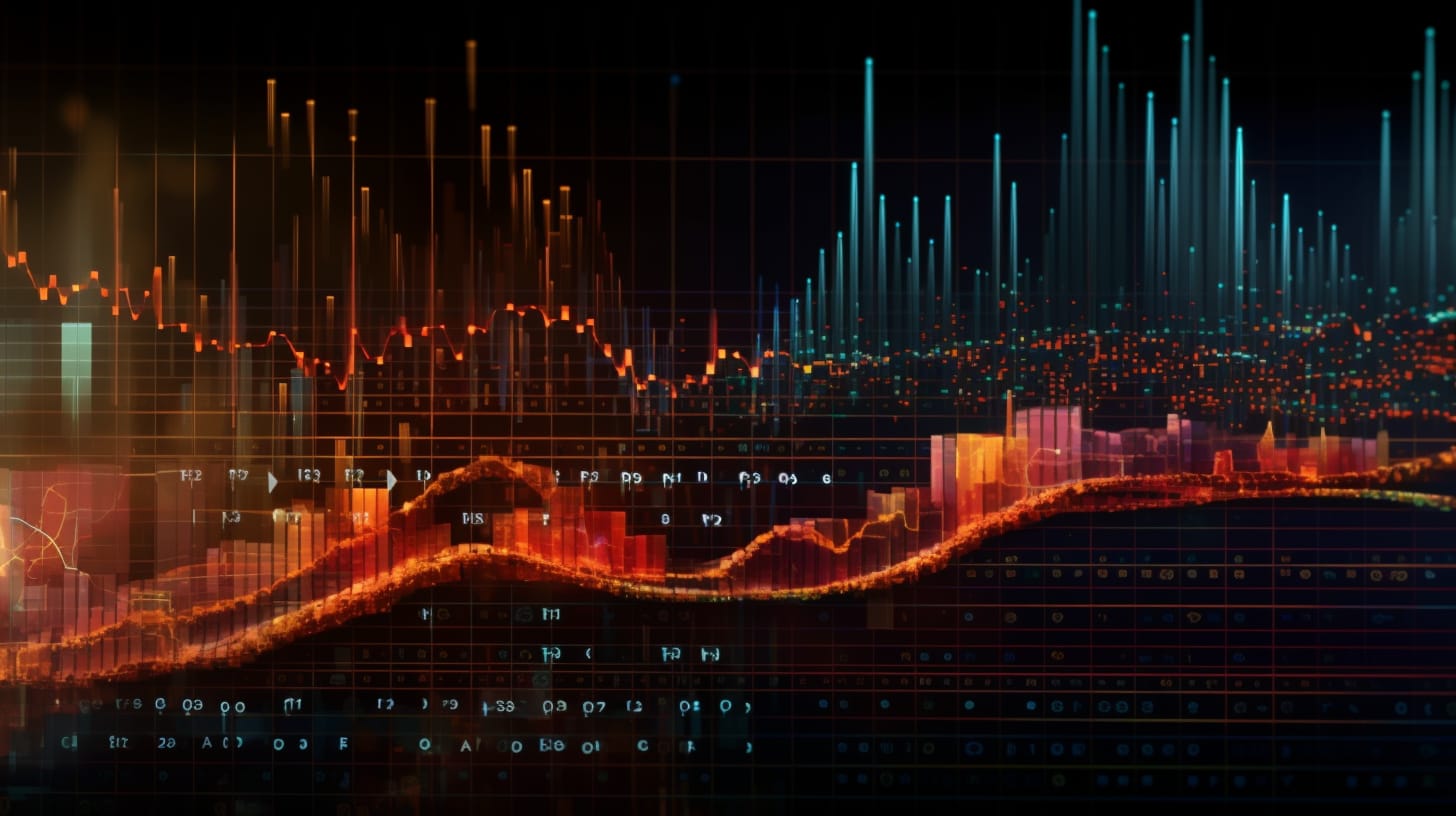,Machine learning is a rapidly evolving field, with new developments and trends continually emerging. As research focuses on developing more general applications and techniques, major vendors are racing to provide platform services, and ethical AI and responsible innovation are becoming increasingly important.,In the near future, we can expect significant advancements in machine learning technologies, as well as new applications and use cases across various industries.,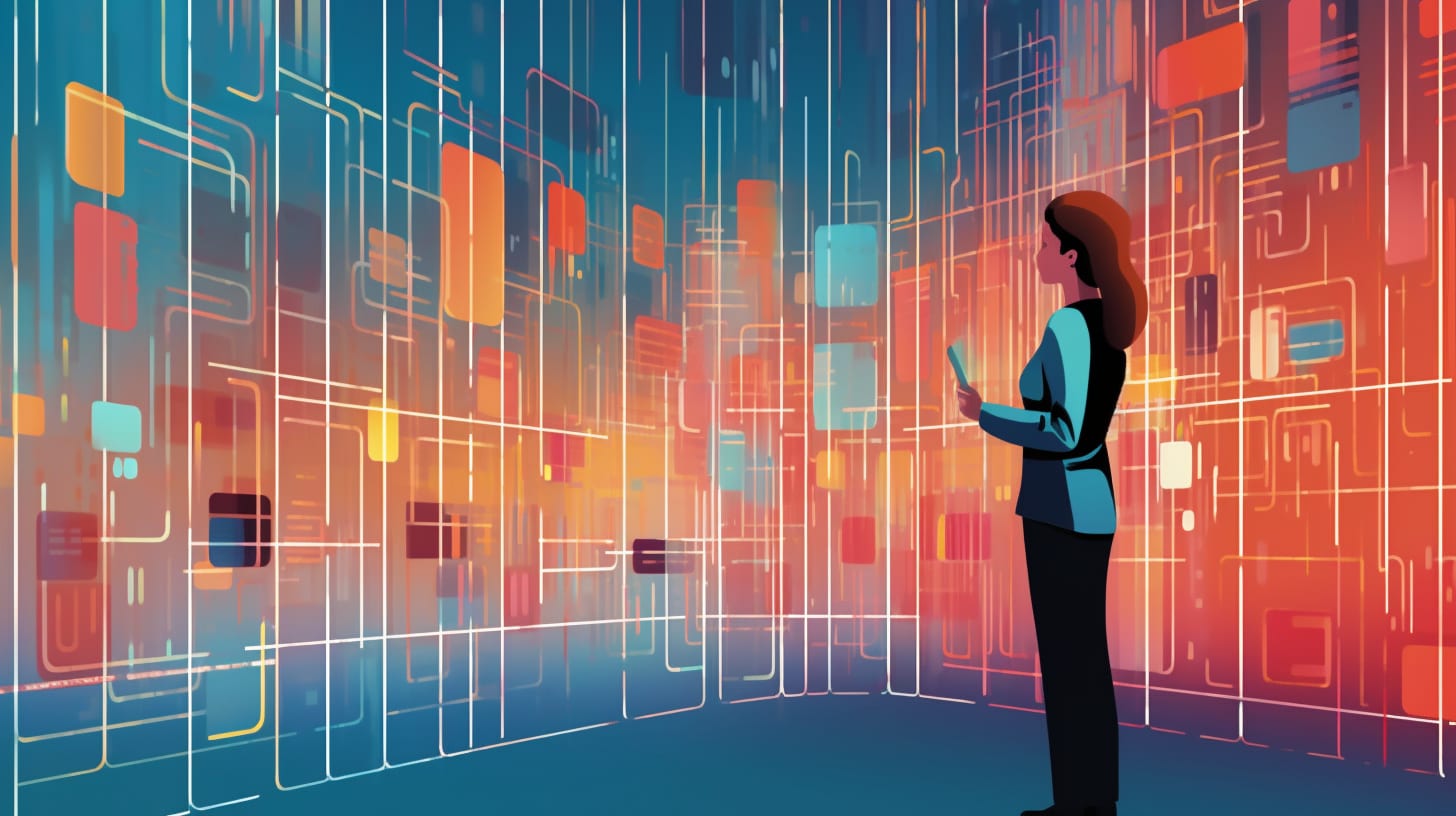,Advancements in machine learning are leading to the development of more efficient algorithms and statistical methods, enabling machines to perform a wider range of tasks with greater accuracy. From image and speech recognition to natural language processing, predictive analytics, and recommendation systems, machine learning is becoming an increasingly powerful tool for businesses and individuals alike.,As machine learning techniques continue to evolve, they are likely to be integrated with other emerging technologies, such as blockchain and the Internet of Things (IoT), further enhancing their capabilities and applications. This convergence of technologies will drive new innovations and create transformative solutions for a wide range of industries.,The growing demand for machine learning solutions has led to increased competition among major vendors, such as Amazon, Google, Microsoft, and IBM, to provide platform services for machine learning and AI developers. These platform services offer cloud-based tools to facilitate the work of data scientists, automate data workflows, expedite data processing, and optimize related capabilities.,As competition intensifies, we can expect further advancements in platform services, making machine learning more accessible and efficient for businesses and developers. This will inevitably lead to the development of new machine learning applications and solutions, pushing the boundaries of what is possible with this powerful technology.,As the utilization of machine learning across various industries grows, ethical AI and responsible innovation become ever more critical. Ensuring that AI is employed responsibly and ethically is essential to guarantee that it does not lead to discrimination against certain groups or individuals, and that its development is secure, humane, and environmentally conscious.,Solutions to ethical AI in machine learning involve using representative and unbiased data sets, deploying transparent and accountable algorithms, and employing techniques such as explainable AI to guarantee that the decisions made by the algorithms are comprehensible and interpretable.,By addressing these challenges and fostering a culture of ethical AI and responsible innovation, we can ensure that machine learning continues to have a positive impact on society and our daily lives.,Machine learning has come a long way since its inception in the 1950s, transforming industries and reshaping the way we live and work. From personalization and recommendations to healthcare and pharmaceuticals, machine learning is unlocking new possibilities and driving innovation across various sectors. While challenges and ethical considerations must be addressed, the future of machine learning is promising, with advancements in general applications, platform services, and ethical AI set to reshape our world.,As we continue to explore the potential of machine learning, it is crucial to embrace a responsible and collaborative approach to ensure its benefits are realized by all. By understanding the basics, implementing effective solutions, and staying informed about future trends and developments, we can unlock the power of machine learning and harness this transformative technology to create a better and more connected world.